Proxy-Powered Insights: Analyzing the Varied Dimensions of Data Wrangling and Data Cleaning
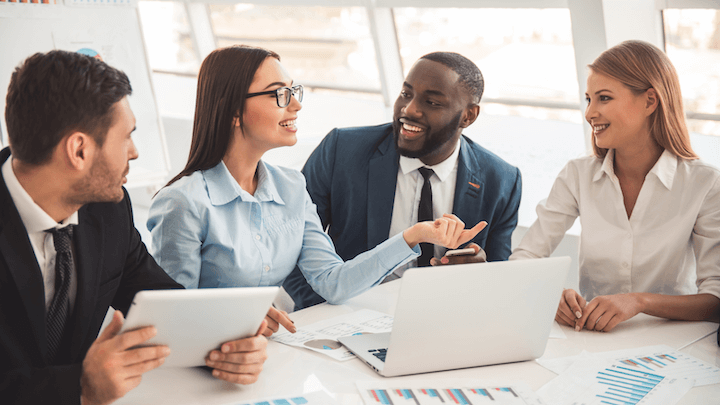
Data wrangling and data cleaning are indispensable cornerstones in the ever-expanding landscape of data-driven decision-making. As organizations grapple with vast and heterogeneous datasets, the significance of refining raw information into accurate, usable insights cannot be overstated.
Intrinsically linked to the integrity of analytical outcomes, data wrangling and cleaning are pivotal for ensuring reliable and meaningful results. The consequences of neglecting these phases can range from misinformed strategic decisions to compromised business operations.
So, we unravel the potential of proxy-powered strategies to revolutionize data wrangling and cleaning, fostering a new era of data accuracy and analytical efficiency. Get ready for insights!
The Significance of Wrangling and Cleaning
These form the foundation of a reliable analysis. This section delves into their critical role in ensuring accurate insights and decision-making.
π¨βπ» Challenges posed by raw and messy data in analytics
The digital landscape is often complex, presenting challenges that hinder the seamless extraction of meaningful insights. Raw and messy data, characterized by inconsistencies, duplications, and incompleteness, can thwart analytical efforts. Navigating through this data jungle demands meticulous data preparation to transform it into a coherent, usable format.
π¨βπ» Flawed insights arising from inaccurate or incomplete facts
Inaccurate or incomplete info can cast doubt over the validity of analytical outcomes. The repercussions of working with subpar info extend beyond mere inconvenience, potentially leading to erroneous conclusions and misguided strategies. Key issues include:
- Bias in analysis: Incomplete info can introduce biases, skewing results and leading to inaccurate interpretations.
- Misleading trends: Anomalies or missing facts can distort trends and patterns, leading to misjudged projections.
- Diminished predictive power: Fluctuations or gaps may weaken predictive models’ efficacy, reducing reliability.
- Compromised decision-making: Relying on inaccurate facts can result in suboptimal business decisions, hindering growth and success.
π¨βπ» Real-world examples of poor data quality impact
- Financial losses: A retail company’s flawed inventory records led to overstocking, causing financial losses due to excess storage costs and unsold items.
- Medical errors: Inaccurate patient records in healthcare settings have resulted in incorrect diagnoses and treatment, posing serious health risks.
- Faulty market insights: Inaccurate customer demographic skewed market analysis, leading to ineffective marketing campaigns and missed opportunities.
Fundamentals You Must Know
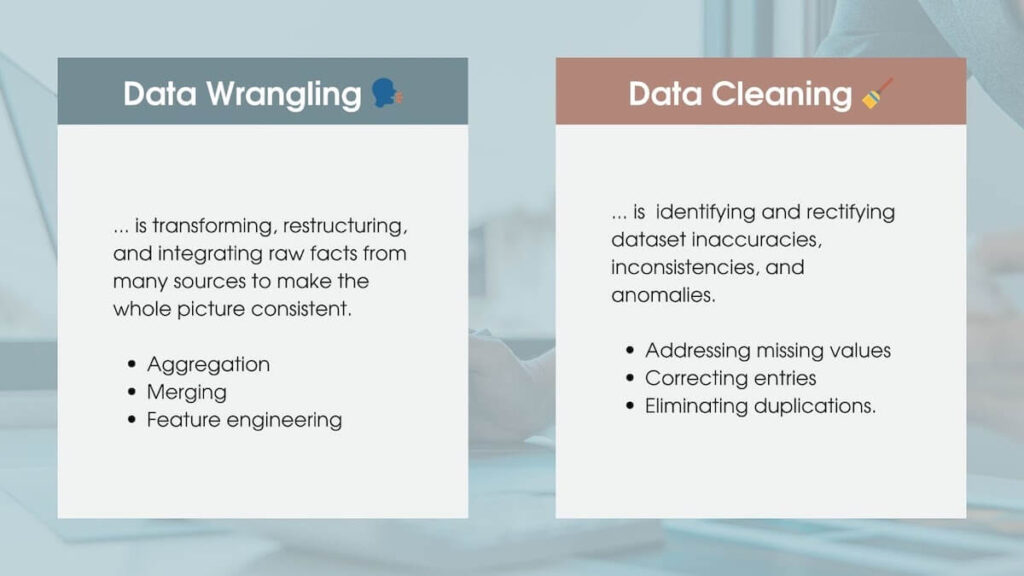
π Defining wrangling and cleaning
- Wrangling involves transforming, restructuring, and integrating raw facts from many sources into a consistent format suitable for analysis. It encompasses aggregation, merging, and feature engineering to create a unified dataset.
- Cleaning focuses on identifying and rectifying dataset inaccuracies, inconsistencies, and anomalies. It involves addressing missing values, correcting erroneous entries, and eliminating duplications.
π Common data quality issues
The journey of information from its raw state to valuable insights is fraught with quality challenges:
- Missing values: Incomplete points hinder analysis and lead to biased results.
- Duplicate records: Redundant entries skew statistics and may inflate the significance of certain patterns.
- Outliers: Unusually extreme values can distort statistical analyses and predictions.
- Inconsistent formats: Differences in formats across sources can hinder integration and analysis.
- Entry errors: Typos, incorrect values, and formatting mistakes introduce inaccuracies.
- Irrelevant information: Extraneous or outdated information can cloud analysis and decision-making.
π Techniques for wrangling and cleaning
Various techniques empower professionals to mitigate the challenges mentioned above:
- Imputation methods: For handling missing values, techniques like mean, median, and mode imputation or more advanced methods like regression imputation can be employed.
- Deduplication: Algorithms identify and remove duplicate records, ensuring accuracy.
- Outlier detection: Statistical methods or machine learning algorithms identify and manage outliers.
- Normalization and standardization: These techniques bring records to a consistent scale, aiding meaningful comparisons.
- Regular expressions: Useful for pattern matching and extraction in unstructured text.
- Validation rules: Implementing rules and constraints to prevent entry errors.
- Automated cleaning tools: Leveraging software to streamline and expedite the cleaning process.
A New Approach: Proxy Variables
Integrating proxy variables introduces a transformative dimension in an era of innovation.
Proxy, surrogate, or indicator variables serve as stand-ins for complex or difficult-to-measure attributes. They offer an alternative, indirect means of representing underlying characteristics, facilitating analysis when direct measurements are impractical or unavailable. These bridge the gap between what is readily measurable and what is ultimately of interest, enabling practitioners to navigate intricate digital landscapes.
π» Leveraging surrogates
Proxy variables bring a novel approach to wrangling and cleaning by aiding in identifying and resolving quality issues. Their application is two-fold:
- Quality assessment: Surrogates can indicate data quality, revealing potential anomalies or inconsistencies that might go unnoticed. An example is using the consistency of timestamps as a proxy for entry accuracy.
- Missing data imputation: In scenarios where missing data is prevalent, indicator variables can be employed to estimate missing values. For instance, using demographic information to predict missing customer purchase data.
π» Examples
The power of proxy-powered insights is evident in practical use cases:
- Environmental monitoring: Weather patterns can serve as proxies for broader ecological shifts in analyzing environmental changes, enabling informed decision-making without directly measuring every aspect.
- Economic indicators: Indicator variables like retail sales data can provide insights into overall economic health, informing policy decisions even when comprehensive economic data is lacking.
- Healthcare analysis: Using readily available vitals (like heart rate and blood pressure) as proxies for overall health status streamlines patient monitoring and assessment.
These streamline the process, enhancing accuracy and efficiency. By ingeniously tapping into alternative sources, practitioners can uncover hidden patterns, detect anomalies, and impute missing values more effectively. This innovative approach not only expedites the preparation of data for analysis but also enriches the quality of insights drawn from that information, propelling the field of analysis into a new era of efficiency and effectiveness.
Advanced Techniques: Machine Learning and Automation
The evolution of analytics has witnessed the integration of advanced techniques, such as AI machine learning and automation. Let us look at the profound impact of these innovative approaches, highlighting their roles, challenges, and invaluable contributions.
Machine learning has been a potent tool for data quality. By harnessing the prowess of algorithms, practitioners can identify and rectify subtle quality issues that evade traditional methods. Anomalies, outliers, and patterns often elude human detection and become discernible through machine learning-driven anomaly detection and clustering techniques. These algorithms autonomously learn from patterns, enabling the pinpointing of discrepancies and irregularities that could distort analysis outcomes.
Automation, powered by machine learning and complemented by indicator variables, further revolutionizes work with information. Proxy variables, acting as reliable guides, enable automation systems to make informed decisions when handling missing data, imputing values, or flagging anomalies. Automation expedites the process and enhances consistency by eliminating human errors. With increasing info volumes, the application of automated systems becomes indispensable for maintaining data integrity at scale.
However, integrating advanced techniques is full of challenges. Machine learning requires extensive data preprocessing and fine-tuning, demanding expertise and computational resources. The interpretability of machine learning models can also pose a hurdle in understanding and validating their decisions. Automation, while efficient, necessitates careful design to ensure indicator variables accurately represent the underlying attributes. The benefits, however, far outweigh these challenges.
The Best Practices for Implementing Proxy-Boosted Insights
That requires thoughtful planning and execution to maximize their efficacy. It is high time to outline key best practices for harnessing the potential of indicator variables in detail wrangling and cleaning.
π Selecting appropriate indicator variables
Selecting suitable surrogates is pivotal for accurate and insightful results. Consider the following!
- Relevance: Ensure the proxy variable has a meaningful and verifiable relationship with the target attribute to be inferred.
- Causality: Understand the causal relationship between the proxy and target variables, avoiding situations where correlations might lead to misleading conclusions.
- Availability: Opt for options that are readily available and consistently measured, reducing the risk of incomplete or biased insights.
π Designing and implementing proxy-powered cleaning
Designing an effective proxy-powered material cleaning process involves careful planning and methodology:
- Mapping and validation: Map variables to target attributes and validate their effectiveness through exploratory analysis and domain expertise.
- Thresholds and triggers: Define thresholds or triggers for using variables, ensuring they are applied in appropriate contexts, such as missing data imputation or outlier detection.
- Iterative refinement: Implement an iterative process for refining proxy-powered cleaning techniques based on feedback and continuous monitoring.
π Mitigating pitfalls and challenges
Despite the advantages of such insights, potential pitfalls should be acknowledged and addressed!
- Proxy quality: Inaccurate or irrelevant proxy variables can lead to misguided inferences. Rigorous validation and domain expertise are essential to ensure proxy quality.
- Overfitting: Carefully guard against overfitting, where proxy variables may capture noise rather than true underlying patterns. Regular validation and cross-validation can help mitigate this risk.
- Context sensitivity: Proxy variables may perform differently across contexts. Consider factors such as material sources, timeframes, and external influences that may impact the proxy’s effectiveness.
Successfully implementing proxy-powered insights demands a strategic blend of data expertise, domain knowledge, and careful consideration of variables and processes. By adhering to these best practices, practitioners can navigate the complexities with precision and confidence, unleashing the true potential of proxy-powered analytics.
Future Trends and Innovations
As the analysis landscape evolves, the potential for proxy-powered insights and data cleaning to drive transformative change is profound. Anticipating future advancements and considering emerging technologies can provide valuable insights into the trajectory of these practices.
The evolution of proxy-boosted insights is poised to bring about several notable advancements:
- Enhanced opinion mining: By leveraging proxy variables derived from sentiment analysis or social media materials, organizations can gain deeper insights into public sentiment, facilitating more informed decision-making.
- Deeper prescriptive analysis: Advanced machine learning techniques can enable proxy variables to predict outcomes and prescribe actionable recommendations.
- Temporal analytics: Proxies can unlock richer historical perspectives, enabling more comprehensive temporal analysis and trends over extended periods.
π The merging technologies and methodologies are…
- Automated feature engineering: Machine learning-driven algorithms can autonomously generate relevant features, reducing the manual effort required for feature selection and engineering.
- Blockchain for provenance: Blockchain technology can enhance data lineage and integrity, ensuring traceability and authenticity throughout the wrangling process.
- Natural language processing (NLP) solutions: NLP-powered tools can assist in automating text data preprocessing, making sense of unstructured text more efficiently.
- Federated learning: Collaborative model training across decentralized sources can enable wrangling without centralizing sensitive facts, enhancing privacy and security.
π The evolving role of data scientists and analysts
As proxy-powered insights and advanced techniques become increasingly integrated, scientists’ and analysts’ roles are set to transform!
- Hybrid expertise: Professionals will need a fusion of data science, domain knowledge, and creative thinking to select, validate, and leverage proxy variables effectively.
- Strategic decision architects: Data scientists will be pivotal in selecting and utilizing proxy variables to address specific business challenges and inform strategic decisions.
- Ethical and responsible usage: As the scope of data-driven insights broadens, data scientists and analysts will face new ethical considerations, requiring them to ensure that proxy variables are used responsibly and aligned with organizational values.
Proxy-unlocked insights, combined with emerging technologies and evolving roles, will usher in a new era of precision, depth, and efficiency in data analysis, enabling organizations to extract richer insights and make more informed decisions than ever before.
Final Words
The symbiotic relationship between proxy-powered insights and wrangling shines as a beacon of innovation in the dynamic digital analysis landscape. From unraveling the intricacies of facts quality to paving the way for predictive prowess, this journey has revealed the transformative potential within these processes!
As organizations harness the power of proxy variables, they navigate the complex maze of information with newfound precision, extracting valuable insights from seemingly disparate sources. The amalgamation of machine learning, automation, and domain expertise propels data wrangling into uncharted territory, promising enhanced accuracy, efficiency, and strategic decision-making.
With emerging technologies and shifting roles, the digital landscape will continue to evolve, propelled by the potential of proxy-boosted insights. In this era of limitless possibilities, the future holds a tapestry of insights waiting to be woven, promising a renaissance of discovery and innovation⦠we can enjoy this to the maximum!